Coronary artery disease (CAD) remains the leading cause of death worldwide in both men and women, accounting for approximately 16% of the world’s total deaths in 2019 alone. Fortunately, with proper diagnosis and management, patients with CAD can be appropriately treated.
Computed tomography-derived fractional flow reserve (CT-FFR) has demonstrated the potential to improve the diagnosis of patients with CAD. DeepVessel FFR, Keya Medical’s deep learning-based CT-FFR software approved for clinical use in China and the European Union, combines the digital images created during a non-invasive coronary computed tomography angiography (CCTA) exam to estimate fractional flow reserve (FFR) using deep learning algorithms. Recent evidence and clinical trials have demonstrated that combining deep learning with non-invasive CT-FFR detection can help improve outcomes in patients with stable CAD as compared to alternative diagnostic tests.
What is Coronary Artery Disease?
Diagnosing Coronary Artery Disease in Symptomatic Patients
Single Photon Emission Computed Tomography
Exercise Stress Test
Exercise stress testing is commonly used for the detection of CAD in patients presenting with chest pain, and it offers a less expensive pathway to diagnosing CAD. However, according to research published in The International Journal of Clinical Practice, the diagnostic accuracy of exercise stress testing depends on factors including the age, gender, and clinical characteristics of the patient [2]. Additionally, exercise stress testing is more useful at excluding CAD rather than confirming that a patient has it.
Stress Echocardiogram
Invasive Coronary Angiogram
According to a study published in the American Heart Journal, only 41% of patients referred for elective ICA are found to have obstructive CAD [4]. This exposes patients to greater risk as they undergo unnecessary invasive procedures while incurring additional costs.
Fractional Flow Reserve
Fractional Flow Reserve (FFR) provides clinicians with a tool to conduct functional analysis of the heart. It is widely accepted as the gold standard for assessing the functional significance of stenotic lesions and guiding coronary revascularization procedures [5].
Multiple randomized trials have demonstrated the diagnostic value of FFR. The Fractional Flow Reserve Versus Angiography for Multi-vessel Evaluation (F.A.M.E.) trial illustrated that FFR-guided intervention could improve patient outcomes. In this trial, FFR-guided intervention resulted in a 33% reduction in MACE when compared to invasive coronary angiography [6]. However, current guidelines recommend assessing myocardial ischemia in stable patients with CAD through non-invasive functional testing before considering invasive coronary angiography or conducting myocardial revascularization [5,7].
Coronary Computed Tomography Angiography
Coronary computed tomography angiography is a non-invasive imaging test used to examine the arteries that supply blood to the heart. During a CCTA procedure, contrast material is injected into a vein in a patient’s arm and a computed tomography scanner is used to take pictures of the heart vessels from outside the body. This allows clinicians to obtain 3D images of the heart and vessels that can be used to observe, measure, and characterize plaque buildup in the coronary arteries and determine if there is any vessel narrowing.
Recent clinical trials suggest that CCTA should be used as a first-line diagnostic test for evaluating patients presenting with stable chest pain. The Scottish Computed Tomography of the Heart (SCOT-HEART) trial and the Prospective Multicenter Imaging Study for Evaluation of Chest Pain (PROMISE) trial have both shown that CCTA can lead to more appropriate use of ICA while providing better information than functional testing in patients who have stable chest pain with a low burden of obstructive CAD [8,9]. Additionally, the Randomized Evaluation of Patients with Stable Angina Comparing Utilization of Noninvasive Examinations (RESCUE) trial demonstrated similar outcomes between patients who underwent CCTA and patients who underwent SPECT but demonstrated that CCTA was a better predictor of major adverse cardiovascular events (MACE) and revascularization [10].
In response to this mounting body of evidence supporting the usage of CCTA, professional societies including the National Institute for Health and Care Excellence (NICE), European Society of Cardiology (ESC), American College of Cardiology (ACC), and the Society of Cardiovascular Computed Tomography (SCCT) have published new guidelines and recommendations on the first-line usage of CCTA in clinical practice. These guidelines recommend that clinicians adopt a CCTA-first strategy when diagnosing CAD in patients with stable chest pain in whom obstructive CAD cannot be excluded by clinical assessment alone.
DeepVessel FFR: Deep Learning-based CT-FFR
Combining CT-FFR with deep learning technology offers an alternative, non-invasive way of diagnosing CAD that can overcome the potential limitations of standalone diagnostic tests. DeepVessel FFR performs a non-invasive physiological functional assessment of the coronary arteries and accurately predict FFR values based on CCTA digital images. The software uses deep learning technology to construct 3D models of the coronary arteries and estimate FFR values non-invasively. As compared to traditional FFR techniques, DeepVessel FFR provides clinicians with a more efficient and affordable diagnostic tool to identify potential narrowing of the coronary arteries that may impact blood flow to the heart.
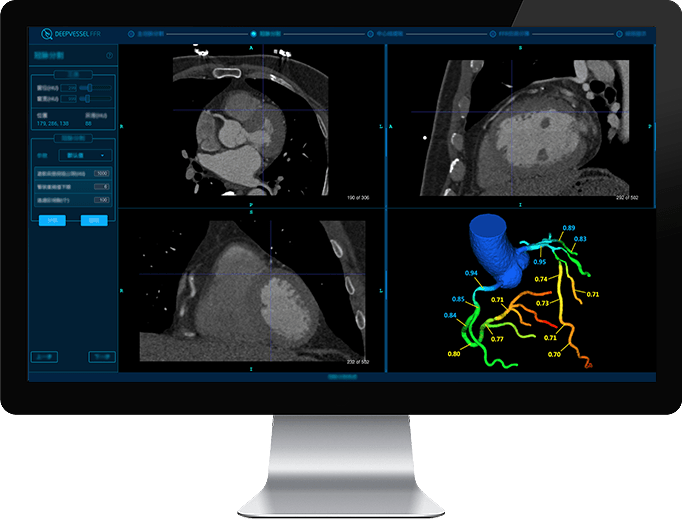
Figure 1: Deep learning-based CT-FFR software, DeepVessel FFR, generates a 3D model of the coronary artery tree and computes non-invasive FFR values from CCTA DICOM images.
Benefits of Deep Learning-based CT-FFR
Multiple studies have investigated the performance of deep learning-based CT-FFR compared to invasive diagnostic tests. A 2019 study published in the Journal of Geriatric Cardiology evaluated the performance of DeepVessel FFR in calculating FFR values from CCTA images using deep learning. The single-center prospective study included 63 patients and the performance of the software was assessed using invasive FFR as the reference standard.
According to the study findings, DeepVessel FFR correlated with invasive FFR, demonstrating that deep learning-based CT-FFR techniques can allow for efficient, non-invasive assessment of the functional significance of coronary stenosis [11].
Researchers at Fuwai Hospital, a leading medical center in Beijing specializing in the treatment of complex cardiovascular diseases, conducted a retrospective study to evaluate deep learning-based CT-FFR in detecting hemodynamic changes of stenosis. The study included 73 patients suspected of CAD who received CCTA followed by invasive FFR within 90 days.
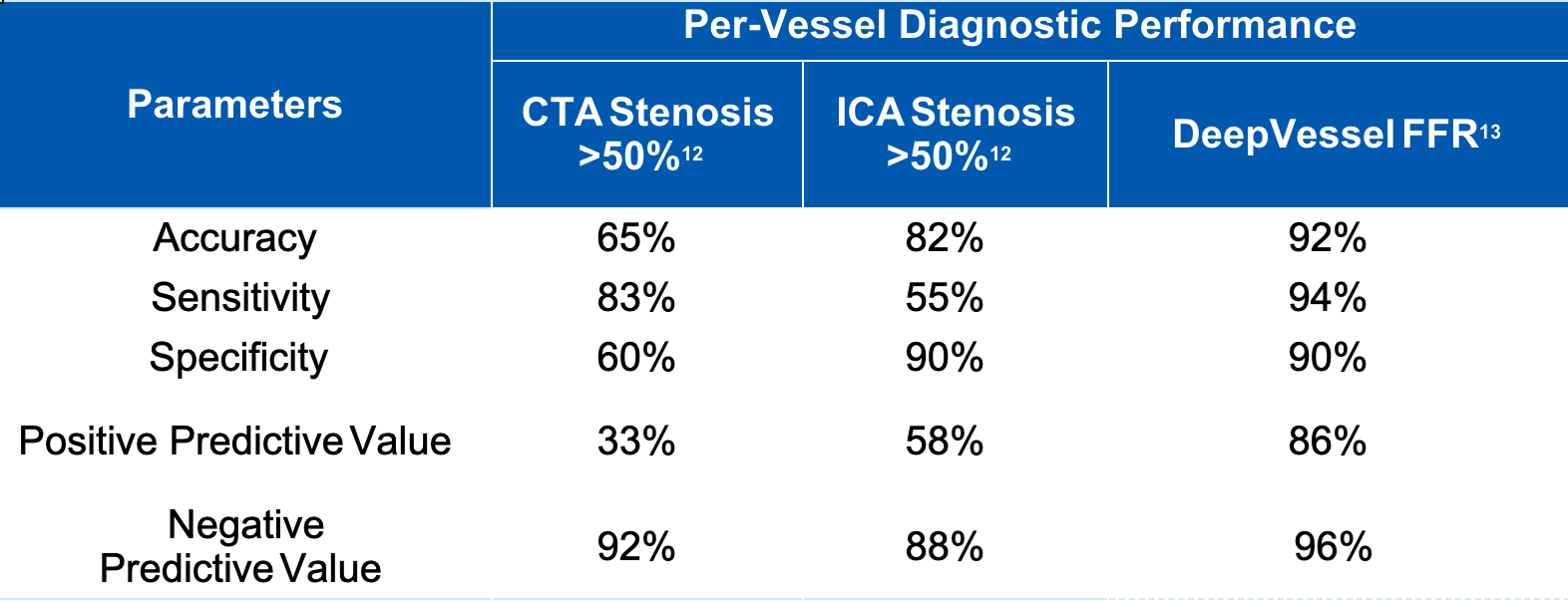
Figure 2: Per-vessel diagnostic performance of DeepVessel FFR as compared to CCTA and invasive coronary angiography alone.
In the study, the performance of CT-FFR exceeded that of CCTA at both the patient and vessel levels while also offering improvements in specificity and positive predictive value without losing sensitivity and negative predictive value. Additionally, the study findings demonstrated that CT-FFR predicted clinical outcomes of patients, including MACE and re-hospitalization. As depicted in Figure 2, DeepVessel FFR achieved an accuracy of 92%, a sensitivity of 94%, and a specificity of 90%, exceeding that of both CCTA and ICA [13].
A separate study conducted by researchers at the First Affiliated Hospital of Jinan University published in European Radiology investigated the use of deep learning-based CT-FFR as an alternative to invasive coronary angiography in the selection of patients for coronary intervention. A total of 243 patients with symptomatic CAD were included in the study.
The study results showed that up to 72% of invasive diagnostic procedures could have been avoided by using a DL-CTFFR value greater than 0.8 as a cut-off for intervention without increasing MACE [14]. The study also showed that revascularization for symptomatic CAD patients guided by the combination of CCTA and DL-CTFFR was associated with a MACE rate like that when guided by coronary angiography in a 2-year follow-up investigation.
Enabling Better Diagnosis & Management of Patients with Coronary Artery Disease
Collaborate with Us
[5] Montalescot G, Sechtem U, Achenbach S, et al. 2013 ESC guidelines on the management of stable coronary artery disease: the Task Force on the management of stable coronary artery disease of the European Society of Cardiology. Eur Heart J 2013;34:2949–3003.
Recent Comments