Hao-Yu Yang
Deep Learning Scientist
Chest radiography or Chest X-ray (CXR), is one of the most powerful and commonly used imaging modalities in clinical settings. It is often the first step in diagnosing conditions within the thoracic cavity. With the recent booming advancement in computer vision and artificial intelligence, automated detection of diseases using CXR has drawn massive attention. In this blog post, let us take a detailed look at two of the largest public Chest X-ray datasets as well as future trends of deep learning developments in this application.
It is difficult to discuss deep learning and Chest X-rays without mentioning the CheXNet. The name originally comes from the Stanford paper “CheXNet: Radiologist-Level Pneumonia Detection on Chest X-Rays”. CheXNet is a Convolutional Neural Network (CNN)-based model that is able to classify a chest X-ray image into one of the fourteen common pathology. Since then, many have adopted “CheXNet” as a general name for applications of neural networks on Chest X-ray diagnosis.
Data plays a role just as crucial as the deep learning modeling in this data-driven era. ChXNet was trained using the Chest X-ray 14 (CXR14) from NIH Clinical Center, one of the first large-scale, publicly available Chest X-ray dataset. It contains a total of 108,948 frontal view Chest X-ray images from 32,717 unique patients. The dataset includes 14 different thoracic pathology labels obtained from text mining on clinical reports. The text mining tool, NegBio, extracts 14 frequent chest radiography observations from free-form radiology reports.
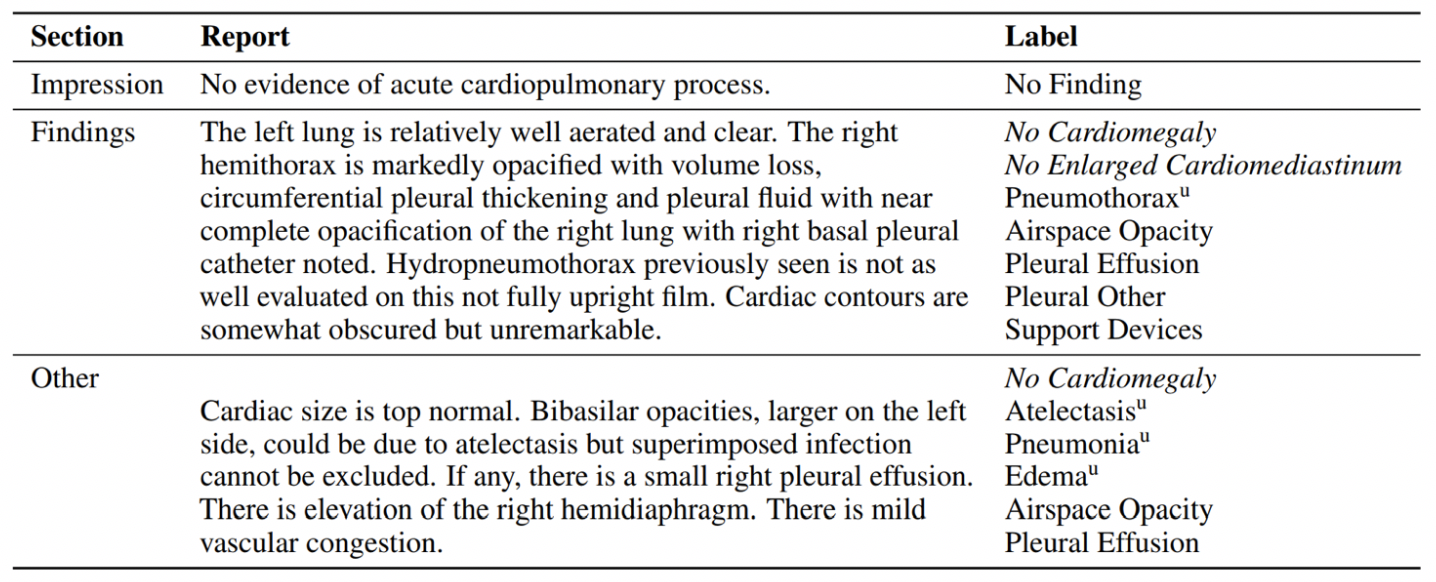
Figure 1: Example of label extraction from a semi-structured radiology report
We have developed a set of in-house algorithms for automatically classifying Chest X-rays here at CuraCloud. Our algorithm is accurate and fast, requiring less than a half a second for a single subject prediction and achieved an average accuracy of 84% across 14 thoracic diseases. The algorithm also provides the area most indicative of the suspected disease, which can help radiologists or physicians understand the model’s “thought process” leading up to the decision.
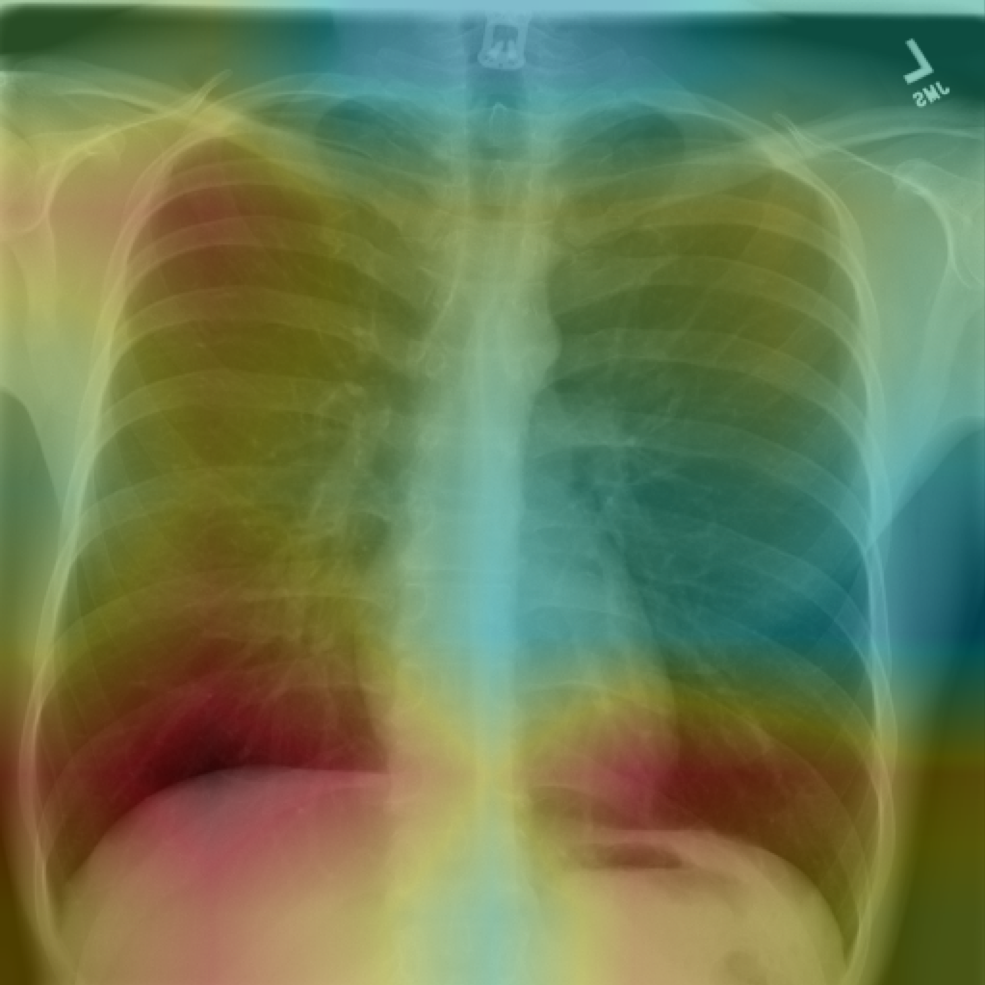
Figure 2: Attention heat map from our in-house CXR detection algorithm
Despite the wide adaption of CXR14 in the AI community, there has been growing concerns regarding the labeling and imaging quality of the dataset. In short, the issues can be summarized as follows:
- Labeling Method: the accompanying labels are extracted from free-form radiology reports using a Natural Language Processing (NLP) tool. Potential error can occur from both the extraction process and the inherent error of using text to describe images
- Image Quality: the pixel intensities range from 0 to 255 in the images provided in CXR-14. However, clinical Chest X-rays typically have intensity levels between 0~3000. The loss of information may cause some lesion hard to recognize or not identifiable at all
- Sample Overlapping: though the number of images may seem large at first glance, images from patient who underwent over 10 scans made up nearly half the dataset. This indicates that there is major overlapping between images and subject level variability may be an issue
This resource was first published prior to the 2020 rebranding of CuraCloud to Keya Medical. The content reflects our legacy brand.
Recent Comments