A screenshot from Dr. Ronald Summer’s presentation at the FDA Public Workshop on the Evolving Role of Artificial Intelligence in Radiological Imaging on Feb. 22, 2020.
The FDA invited speakers with varied perspectives, including NIH labs, practicing radiologists, professional association representatives, and patients. The practical benefits of applying AI-enabled computer vision to radiological imaging were clear in many of the presentations from the NIH and commercial developers of medical AI software devices.
Three related themes emerged from the presentations and panel discussions. This includes:
- The FDA’s concern that performance of medical devices should be generalizable across the US population and care settings;
- Training data and problem of “domain shift” in which an AI software medical device that has been trained on one population or in one care setting is used with a new setting, potentially resulting in suboptimal performance;
- The ability to make local adjustments—also known as “localization”—to a generalized AI system to improve its performance with a new population, care setting, or intended use. Federated learning may be a way to balance the needs for generalized and localized performance optimization.
Generalizability
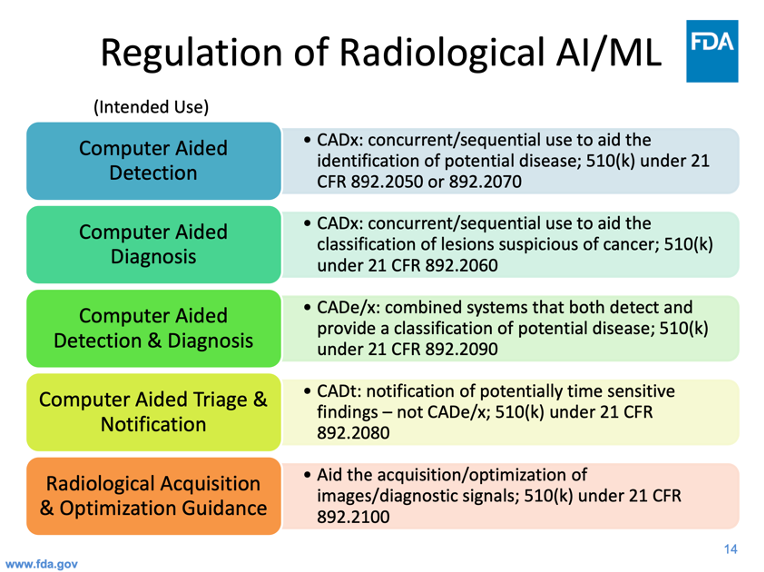
Figure 1: Dr. Ochs’ presentation provides insight into the approach the FDA takes to regulate radiological AI and machine learning devices.
Robert Ochs, Deputy Director of the FDA Center for Radiological Health, provided an overview of the current regulatory landscape for AI and machine learning in radiological imaging. The approach the FDA takes in regulating radiological imaging AI has a profound influence on commercial availability of products, and consequently on the product strategy of software device manufacturers. Because computer aided detection (CADe) and computer aided diagnosis (CADx) applications were considered Class III until Jan. 2020, the burden of proof of clinical effectiveness was very high, resulting in relatively few approvals. Meanwhile, advances in deep learning and the popularity of data science competitions were mobilizing thousands of scientists who produced working CADx models. The agency created a new Computer Aided Triage and Notification product class (CADt) which now, according to one speaker, accounts for most of the current wave of startup activity.
Dr. Ochs explained some of the issues facing regulators now that technology advances are increasing the feasibility of autonomous devices. He shared that:
“…. We’re going to promote best practices in the study designs that match the intended use and indications. We want to avoid seeing — tuning in to the validation data set, which is a common mistake that we see. The challenges are assessment of the generalizability and the true match the intend use and indications want to avoid tuning to the validation data set which is a common mistake that we see. The challenges are assessment of the generalized robustness of the system and want to think about the pre-specification for algorithm changes and testing protocols and seek greater insight into premarket performance and a lot of this involves the thoughts you heard earlier about digital health efforts how to regulate the devices adjust for continuous changes, and do so in a way that’s practical and helpful for our mission.”
The agency has recently begun to approve certain autonomous AI devices capable of determining which images are normal and which require further review. These devices are not without precedent. In 1995, the FDA approved an automated cervical cytology slide reader, BD FocalPoint GS (P950002-S002). More recently the agency approved a device for autonomous detection of diabetic retinopathy (IDx-Dr).
Training Data Enhancement and Domain Shift
“So, for instance, a developer has a cleared algorithm that he brings to a site. The performance is not as good as was in the parameters specified in their FDA clearance so what happened is that they end up training it on a hundred, a thousand, some number, of cases at the site. The performance of the algorithm improves, of course, biased to their site, but so what, that’s where you want it to work the best, right?”
Localization and Federated Learning
“Next we have more federated machine learning approach, so rather than a single central learner that learns simultaneously across sites we take the learning algorithm and pass it around to different potential collaborators.
This model itself is again very interesting to think about because you can imagine that during deployment… rather than giving you an institution a fully trained 100 percent algorithm I can give you a federated model that’s been trained 90 percent of the way there and your specific institution is simply the final node in that federated model. You’re the last person to update the weights.
Every institution in that case may have their own unique model to work with. Sort of the hyper-optimization mentioned this morning so certainly a very feasible and interesting approach.”
Asking the Right Questions
“… while today we see that most of that algorithm development may be done in commercial entities, I see that in the very near future academic hospitals or university departments will take it upon themselves more and more to be building home grown solutions. In fact, to the point where I imagine there will be a rapid blurring between what we often times consider as a research project at a single institution, versus full clinical deployment in the hospital. And so certainly the question here is what the scope of potential regulatory considerations might be, will the regulatory burden be placed on companies whose job is to curate and aggregate models from different academic hospitals, will it in fact be on the very specific institution if they are producing many models that a lot of different hospital are using.”
This resource was first published prior to the 2020 rebranding of CuraCloud to Keya Medical. The content reflects our legacy brand.
Recent Comments